|
ACCESS THE FULL ARTICLE
No SPIE Account? Create one
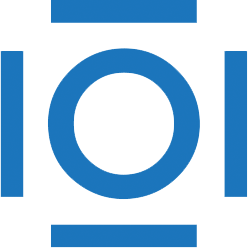
CITATIONS
Cited by 2 scholarly publications.
Tumors
Brain
Magnetic resonance imaging
Image segmentation
Binary data
Feature extraction
Tissues